In combination with real images, synthetically generated images ensure greater safety and comfort when driving. To allow modern interior sensing, Bosch Research relies on artificial intelligence.
Things can get quite hectic inside a car: Bored children in the back may unbuckle their seatbelts, toys are thrown around and changing lighting conditions can divert attention away from the road. It can be difficult for drivers to keep on top of everything that’s happening. Interior cameras can help here. They recognize people inside the vehicle as well as their behavior so that they can intervene and lend support.
One such camera is the so-called Occupant Monitoring Camera (OMC), which the Bosch Cross-Domain Computing Solutions division is working on. It adds new functions to the Driver Monitoring Camera (DMC). Developed by Bosch, the camera is already used as standard in vehicle interiors from various manufacturers. The OMC observes and recognizes the entire interior of a vehicle using a special image processing algorithm. The algorithm has been taught by machine learning to understand and analyze the observed scenario and to issue warnings and instructions based on the findings.
The OMC has a wider field of vision than the DMC and recognizes the driver as well as the front passenger and other vehicle occupants. The OMC records how many people are in the vehicle, where they are and what they are doing, and whether the driver could be distracted. This is essential for automated driving. For example, if the driver is tired or in the middle of a conversation, in the future the automated system will not trigger a handover maneuver back to the driver. Instead, the vehicle will come to a stop at the side of the road.
Many road accidents are the result of human error. If the person behind the wheel momentarily nods off, this can have fatal consequences. After all, the vehicle is virtually driverless during this time, even if only for a few seconds. Technology can ensure greater safety here. The EU Commission has also recognized this and made additions to the EU’s “General Safety Regulation” (GSR). This sets out regulations on safety technologies and design features for the transportation of people and goods with vehicles on public roads. One important point: From July 2024, all new vehicles must have a system for detecting driver fatigue. In addition, a system that detects driver distraction will be compulsory from July 2026. With the 2026 protocol, NCAP Vision 2030 is strongly focused at present on passive safety issues, especially in relation to occupant classification and recognizing people in unusual positions. As a result, the importance of pose detection, especially a high-precision 3D pose, is growing once more.
The new functions of the OMC are part of extended interior sensing and can support the sensor systems in the vehicle even more precisely with the information from the interior. They improve safety when driving and meet the EU specifications.
Because the OMC recognizes the people in the vehicle, personalized settings can also be made automatically. For example, the seat can be adjusted specifically for the detected person, the on-board electronics can be individually adjusted or the desired entertainment program can be activated without having to enter it again.
The Bosch Research team headed by Bernd Göbelsmann, Project Manager and Research Engineer, and Dennis Mack, Research Engineer, developed an algorithm for estimating the 3D body pose for the OMC as part of the “AI Methods for Interior Sensing” project. The project serves as a basis for developing applications and camera functions. 3D body pose means that a human body can be recognized in its posture and position in space by the camera algorithm. The estimation of the 3D body pose is carried out on the basis of a neural network. To train the algorithm, you need images — lots of images.
Images showing vehicle occupants in different positions as well as different people, age groups and groupings. “In order for the algorithm to be able to come up with an action from the analysis of the camera images, it should ideally have seen the situation beforehand and must therefore have been trained for this,” explained Bernd Göbelsmann. A data set with real images was created at Bosch Research in 2019. It was produced in a film studio in Hanau with the help of more than 400 people and already represents a good set of basic data. However, such recording campaigns are cost-intensive and time-consuming and it is difficult to recruit the statistically necessary number of test subjects. There is another problem too, one which most people would not expect: There is no depth information in real photographs. “Information such as how far away the people are sitting, the position of a hand inside the vehicle or how far away it is from the camera is not immediately obvious from a camera perspective,” explained Bernd Göbelsmann.
In order to determine this 3D information and obtain suitable training data for the OMC, a depth measurement method such as ToF (time of flight), stereo or multi-camera triangulation is required. ToF camera systems use the time-of-flight method, for example, to measure distances between the camera and the object and create 3D image points on this basis. With a multi-camera system, the 3D image points are calculated using triangulation from several cameras. “We opted for a multi-camera system so that we can also recognize 3D points for hidden persons on the rear seat,” explained Bernd Göbelsmann. Several cameras are used for the initial 3D point determination. The camera algorithm is then trained with a camera on the basis of a camera image and the relevant 3D points.
In order to make the training data set as diverse as possible and avoid the costs of additional real images, the Bosch Research team followed the approach from the outset of supplementing real data with synthetic data. The idea of the research team: To use open source 3D modelling software in order to obtain synthetic training data. This software allows images of various interior scenarios and poses of people to be generated. For the artificial intelligence (AI) on the control unit, i.e. the camera, it is irrelevant whether the image is real or artificial as long as it looks real and it is confronted with statistically sufficient application scenarios and variations as a result. This method saves the development team resources, especially time and money. However, when digitally placing the synthetic persons in the virtual vehicle interior, they can merge with the contour of the seat, for example, and hands or arms may no longer be visible. To prevent this happening in the first place, the Bosch Research team developed an adaptation process: Digital pose correction. Digital pose correction is based on a machine learning process that has learned from human pose recordings which poses can and cannot be assumed by people. Based on this information and knowledge of the position of the vehicle geometry in the virtual scene, the poses are changed so that they do not conflict with the vehicle interior. Care is taken to ensure that the persons’ limbs do not overlap either with themselves or with the limbs of other people and that a realistic pose is nevertheless assumed. This saves the virtual scene designer a lot of manual work and allows poses that could also be assumed in a real vehicle.
However, these AI-generated images are still not sufficiently realistic for training the camera algorithm. The team therefore used generative AI in the next step to create more photo-like training images from the generated images. For the first time ever in this field of research, 3D modeling software was linked with an AI image generator to create training data. The major advantage of this approach is that it reduces the so-called domain gap between synthetic and real data. Domain gap means that the camera algorithm is trained with synthetic images but the OMC ultimately captures and has to evaluate real images. The more photo-like the training data, the more reliable the evaluation of the interior camera used will be. “If only synthetic training is used and the camera model is then tested with real data, the results are worse owing to the domain gap,” said Dennis Mack. As he explained, the edges and silhouettes of the persons along with the textures of bodies or clothing are important here. The same applies to contrasts. What a human eye can recognize in terms of textures and positions must be learned by the AI on the basis of training data. “We assume that the more photo-like the
Numerous and diverse
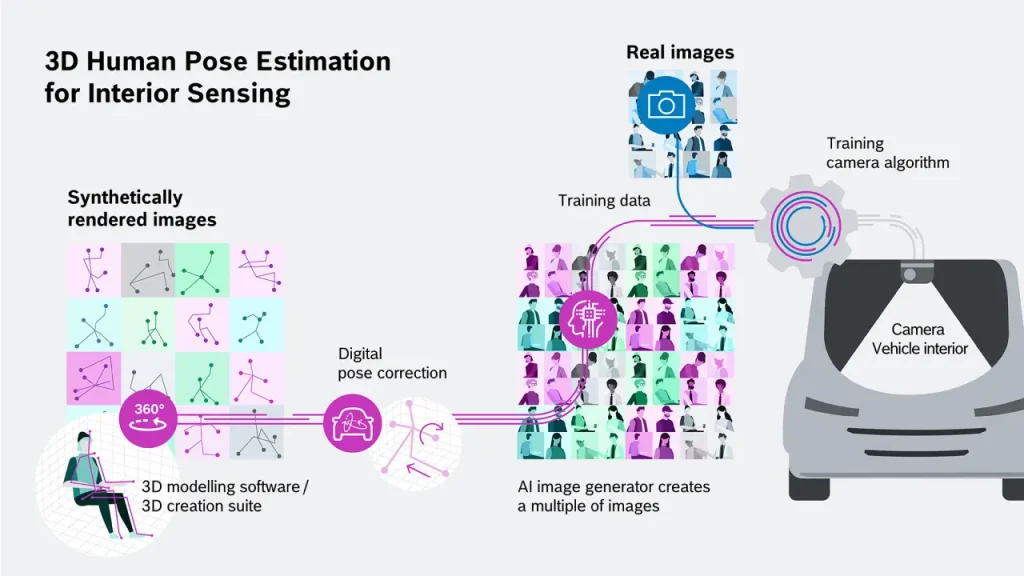
The advantages of synthetically generated data are obvious: The number of people inside a vehicle, their poses, size, age and clothing can be varied quickly and easily in terms of textures. Various vehicle models can also be included in the image simulation. The project team has used around 20 different types so far. Thanks to the modeling software in combination with generative AI, hundreds of thousands of different images can be created in just a few days. It would not be possible to create this number of images in such a short time with recording campaigns of real people and the costs would also be significantly higher regardless of the time factor. After all, when training an image recognition algorithm, the more diverse the situations and poses in the data sets, the better the algorithm can generalize, which means that it also works better in unknown situations.
Another aspect is that synthetic data is consistent with the currently applicable data protection laws since it is not necessary to obtain the consent of a real person in order to use their image data as would be the case with real images.
The future is synthetic
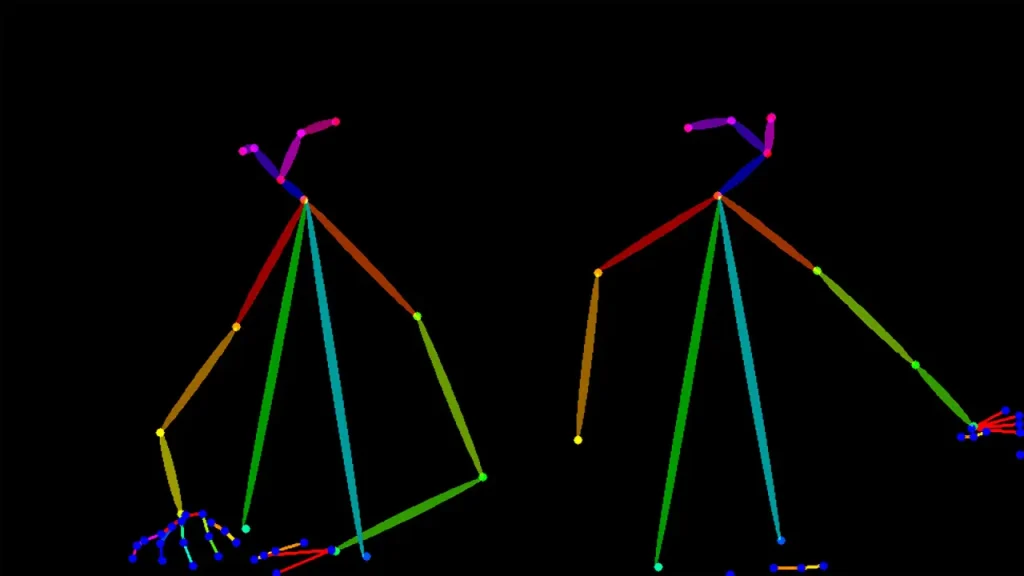
The combination of real images with AI-generated images still delivers the best results at present for training image recognition algorithms. Bosch is also aware of the disadvantages of synthetic data. We analyze these disadvantages carefully and bear them in mind in our products and services. There is usually a domain gap with synthetic data. It may be the case in this project that the synthetic data does not have the same degree of realism as the real data and therefore exhibits different noise patterns to real camera images. The generative approach that is being used for the first time in this project should allow the degree of realism of the synthetic data to be as close as possible to that of real images. In this context, however, synthetic images are still the way forward. With further research, real data may no longer be needed to train camera algorithms.
The Bosch Research project has proven that the use of synthetic data makes sense and allows significant savings. Time-consuming recording campaigns can be avoided thanks to synthetic data and the development time of image recognition algorithms can be significantly reduced – this also applies to other camera types with different installation positions in a variety of vehicle types, for even safer and more comfortable driving.